Why Private infrastructure is better for AI
Time to read: 10 mins
Why Serious Business Projects Using Generative AI Are Better Served with Private Infrastructure
In the rapidly evolving landscape of artificial intelligence (AI), businesses are increasingly leveraging generative AI to drive innovation and efficiency. However, when it comes to serious business projects, the choice of infrastructure – whether on-premises or in a hyperscale public cloud – can significantly impact the security, performance, and control over sensitive data.
Let’s explore why on-premises infrastructure is often the better choice for serious business projects using generative AI.
Running AI Models vs. Building New AI Models: Inferencing and Training
To make informed platform choices depends on a clear understanding of the difference between training a new AI model and running a finished model in the real world (known as inferencing).
Building and training an AI model involves developing the algorithms and feeding them large datasets. This process requires significant computational resources and is often conducted in a controlled environment. Large Language Models (LLMs) are a class of AI models that have been trained on vast amounts of data to understand and generate human-like text. These models, such as GPT-4, excel in a wide range of language-related tasks due to their ability to capture intricate patterns in language. The performance of LLMs is heavily dependent on high computational power, which is why GPUs are essential for training AI applications. High-performance infrastructure ensures that LLMs can operate efficiently, providing accurate and contextually relevant outputs.
Inferencing, on the other hand, is the application of a trained model to make predictions or generate outputs based on new data. This phase is where the model is deployed in real-world scenarios, and its performance is critical to the success of AI-driven projects. On-premises infrastructure is particularly well-suited for inferencing due to its optimised processing capabilities and secure environment.
Enhancing AI Output Quality with Internal Data
Another advantage of on-premises infrastructure is the ability to use internal company-specific data to improve the quality of generative AI output. Retrieval-Augmented Generation (RAG) is a technique that enhances the performance of generative AI models by incorporating relevant information from a company’s internal data sources. This approach improves the accuracy and relevance of the AI-generated content.
However, this benefit comes with its own set of risks. Using sensitive internal data to train AI models can lead to potential data leaks if not properly managed. On-premises infrastructure provides a more controlled environment where businesses can implement stringent security measures to protect their data from unauthorised access and breaches.
Risk of Sensitive Information Leakage
One of the primary concerns with using hyperscale public cloud services for generative AI is the risk of sensitive information being leaked into the public domain. When businesses use public cloud services, they often have to share their data with third-party providers, which increases the risk of data breaches. For instance, if a company uses ChatGPT for generative AI, the final output becomes part of ChatGPT’s base of information, which is then used to train the next version of the model. This means that any sensitive information inputted into the system could potentially be exposed to other users.
A notable example of this risk is the case involving Samsung. In 2023, Samsung employees accidentally leaked confidential company information by using ChatGPT to help with tasks such as fixing source code and converting meeting notes into presentations. This incident highlighted the dangers of using public generative AI services for handling sensitive data, as the information inputted into ChatGPT was retained and used to train the model, making it accessible to others.
Platforms for AI training
IBM Fusion Hyper-Converged Infrastructure (HCI) Appliance
The IBM Fusion Hyper-Converged Infrastructure (HCI) appliance is an ideal platform for running AI on-premises due to its seamless integration of compute, storage, and networking resources. Designed to simplify the deployment and management of AI workloads, IBM Fusion HCI combines the power of Red Hat OpenShift with IBM’s advanced storage solutions. This integration allows businesses to efficiently manage containerised applications and data services, ensuring high performance and reliability. By leveraging IBM Fusion HCI, organisations can maintain control over their sensitive data, reducing the risks associated with public cloud environments while benefiting from the flexibility and scalability of a hybrid cloud approach.
Moreover, IBM Fusion HCI is optimised for AI applications through its support for IBM’s watsonx platform, which enhances AI and data science capabilities. The appliance’s hyper-converged architecture ensures that AI workloads are processed with minimal latency and maximum efficiency, making it a robust solution for mission-critical applications. Additionally, IBM Fusion HCI’s built-in data protection and security features provide a secure environment for AI operations, safeguarding against data breaches and ensuring compliance with regulatory requirements. This makes IBM Fusion HCI a powerful and reliable choice for businesses looking to harness the full potential of AI on-premises.
Platforms for AI inferencing
The Power of IBM Power10 Systems
For businesses looking to optimise AI inferencing, IBM Power10 systems offer a powerful on-premises solution. The IBM Power10 processor includes Matrix Math Accelerator (MMA) units, which are specifically designed to enhance AI inferencing capabilities. These processors provide significant performance improvements over previous generations, making them ideal for running complex AI models in real-time.
The IBM Power10 systems are equipped with Matrix Math Accelerator (MMA) units, which are designed to enhance AI inferencing directly within the processor core. These MMAs handle matrix multiplication operations in hardware, significantly boosting performance for AI tasks without relying on external accelerators like GPUs. By integrating MMAs into each Power10 core, IBM has optimised the system for running AI models efficiently, reducing latency and improving throughput. This setup allows AI applications, such as those using PyTorch and TensorFlow, to leverage these accelerators for faster and more accurate inferencing. Additionally, the on-chip MMAs reduce the data centre footprint and simplify infrastructure management, making Power10 a powerful and cost-effective solution for businesses deploying AI at scale.
The Power10 systems allow businesses to perform AI inferencing directly on-premises, reducing the need for external accelerators and minimising latency. This setup not only ensures faster and more efficient AI processing but also keeps sensitive data within the company’s secure infrastructure, mitigating the risk of data breaches associated with public cloud services.
Role for Public Cloud – R&D vs. Production
Public clouds offer an ideal environment for research and development (R&D) of generative AI projects due to their flexibility, scalability, and cost-effectiveness. During the R&D phase, businesses often need to experiment with different models, datasets, and configurations to find the optimal solution. Public cloud platforms provide the necessary computational resources on demand, allowing researchers to quickly scale up or down based on their needs. This flexibility is crucial for iterative experimentation and rapid prototyping, which are essential components of the R&D process. Additionally, public clouds offer a wide range of AI tools and services, such as pre-trained models and machine learning frameworks, which can accelerate development and innovation.
However, when it comes to moving generative AI projects into production, the considerations shift significantly. Production environments require robust security, compliance, and performance guarantees that are often better managed with on-premises infrastructure. One of the primary concerns is the risk of sensitive information being exposed in a public cloud environment. During production, AI models frequently handle proprietary and confidential data, making data security a top priority. On-premises infrastructure allows businesses to maintain full control over their data, implementing stringent security measures to protect against breaches and unauthorised access. This control is particularly important for industries with strict regulatory requirements, such as finance and healthcare.
Moreover, the performance and reliability of AI applications in production are critical for business operations. On-premises infrastructure can be optimised specifically for the AI workloads of a company, ensuring consistent performance and minimising latency. This is especially important for real-time applications where delays can impact user experience and operational efficiency. By keeping AI operations on-premises, businesses can also avoid potential downtime and service disruptions that might occur with public cloud providers.
In summary, while public clouds are excellent for the exploratory and experimental phases of generative AI projects, on-premises infrastructure provides the security, control, and performance needed for reliable production deployment.
Is AI on Your Radar? (We Can Help)
Whether you have a serious AI project, or you want to discover more about AI adoption and your business, get it touch today for a free, no-obligation discussion for with one of our specialists.
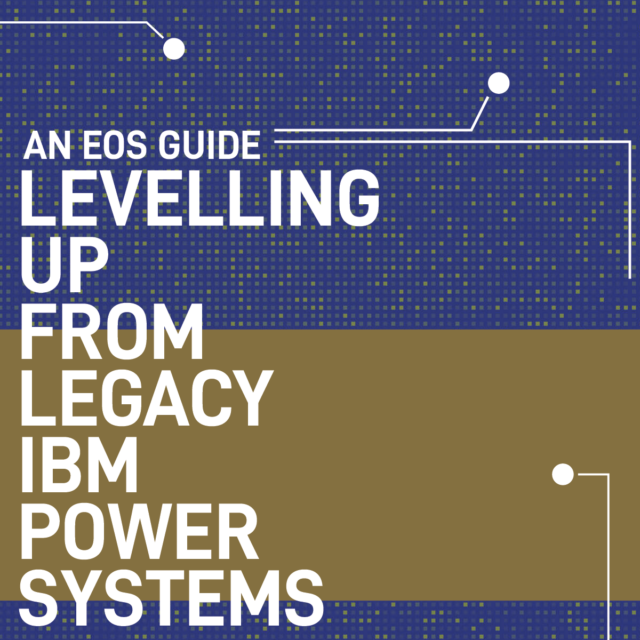
IBM Power
Levelling Up from Legacy IBM Power Systems (An EOS Guide)
Why Modernisation Matters Modernisation is often about market competitiveness and relevance. For modernised organisations, offboarding outdated hardware is a matter…
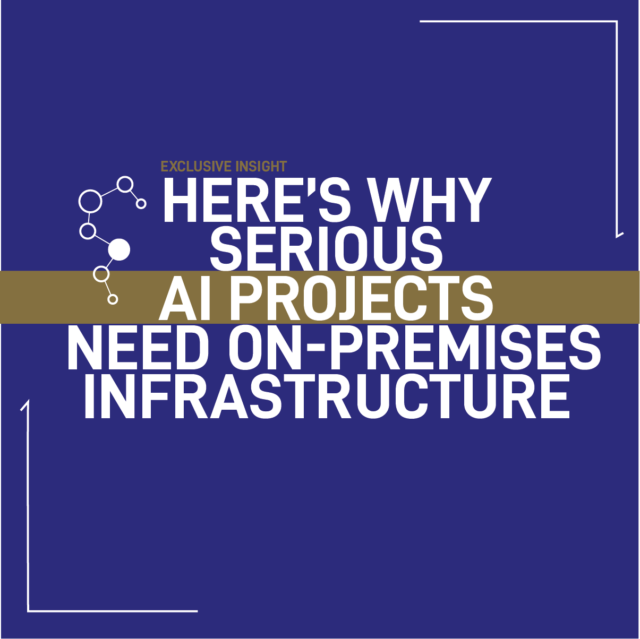
IBM Power
Why Private infrastructure is better for AI
Running AI Models vs. Building New AI Models: Inferencing and Training To make informed platform choices depends on a clear…
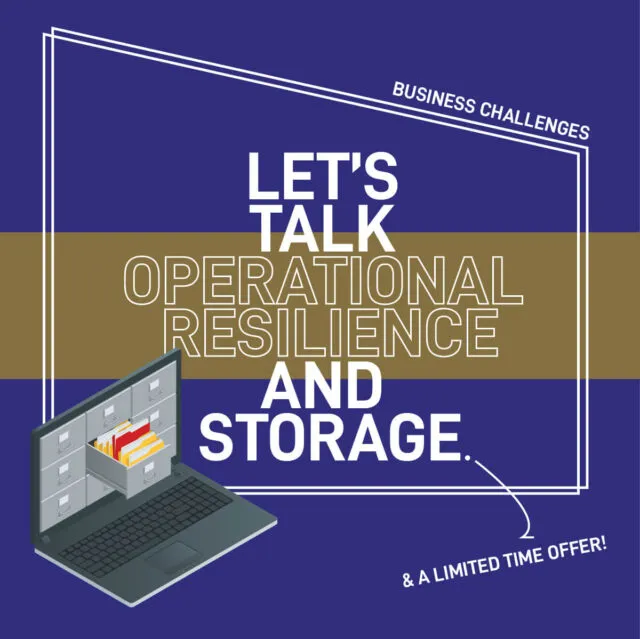
Storage and Backup
Improving Operational Resilience (With AI-driven Technology)
The Financial Impact of Cyber Attacks is Underestimated On average, analysts have discovered, a cyber-attack will force a UK SME…
Ready to talk?
Get in touch today to discuss your IT challenges and goals. No matter what’s happening in your IT environment right now, discover how our experts can help your business discover its competitive edge.